ChatGPT-4o writes a causal inference exam with diff-in-diff, takes the exam, grades the exam, then grades the grading of the exam
Comparing it with the one ChatGP-4 wrote a year ago
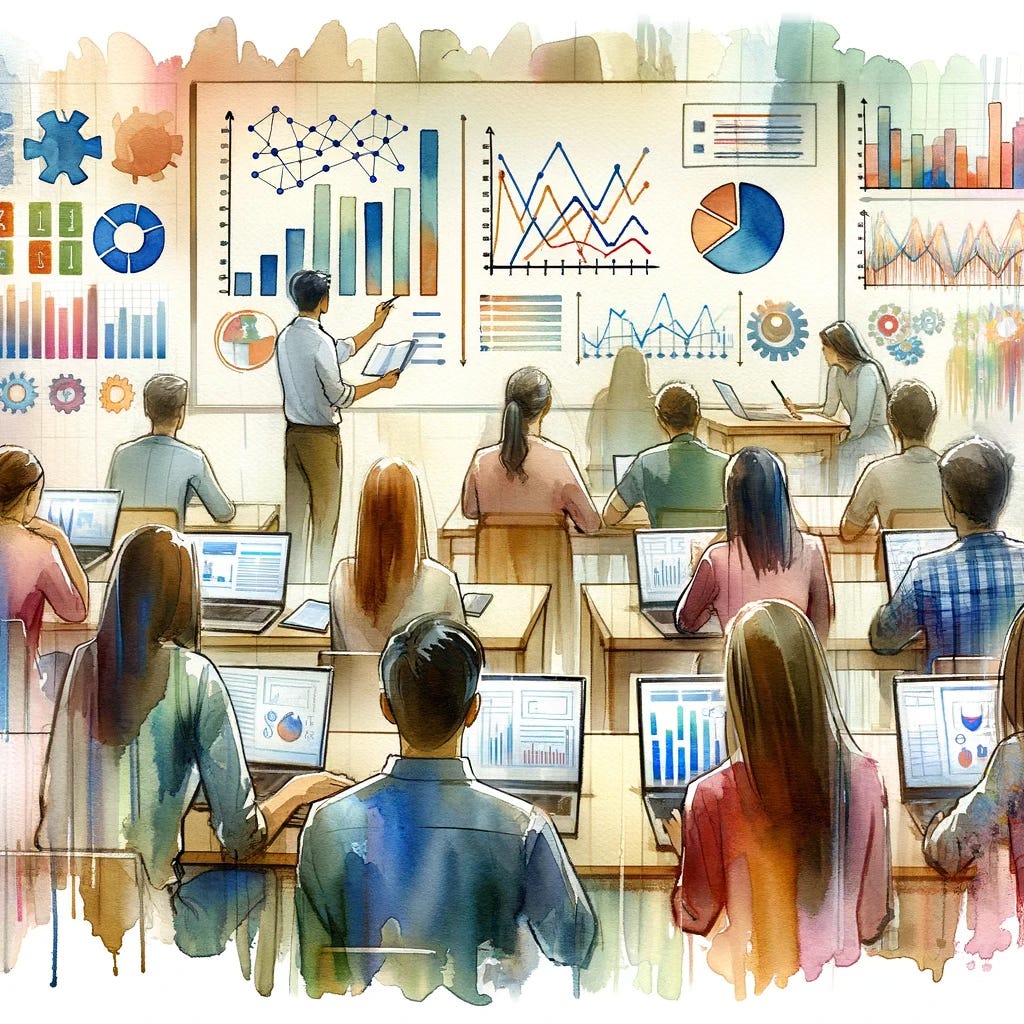
This week, OpenAI announced a slight update to its GPT-4 model called ChatGPT-4o. It’s main updating seemed to be to improve the conversational assistant which is additional more conversational than before (hard to imagine it could’ve gotten much better than it was though), as well as can now interact with a lot more media (like video and images). The video part I think its not out yet for everyone. There will also be an app native to the Mac desktop that I’m excited about, plus ChatGPT-4 and 4o is now free for everyone. I suspect we will be paying for GPT-5 when it comes out, though, as they did this in November 2022 — released ChatGPT-3.5 for free then ChatGPT-4 in March for $20.
Last March, I decided to have ChatGPT-4 write a simple causal inference exam, and then answer the questions, and if you want to see how it did, you can click on this old substack. Today I asked it again, plus I added in some new questions. So let’s compare the old and new answers and have ChatGPT rate how much better the first student did compared to the second one. The only problem will be that the first and second students are looking at different Stata output, but let’s still see if can work around that maybe by using screen shots. I’ll just say that there was a randomized test with different output and slightly different questions.
I’m going to for this post, though, only use difference-in-differences questions. I’ll pretend that I assigned two tests randomly to students, submit the questions and answers it generated back to him, and then ask him to grade them. Then I Will ask which question was harder and why and in light of that which student seemed to have a deeper understanding of the material. Finally, I will about Callaway and Sant’Anna.
First, here is the original exam that ChatGPT-4 wrote back in March 2023. I asked it to both take the exam and answer the exam.
I asked GPT-4 to write three exam questions for a causal inference class, and then take it
Both exams use the same prompts. But each the different ChatGPT-4s wrote different tests and gave different answers. But not surprisingly, both students taking the exam (which were ChatGPT-4 and ChatGPT-4o) got 100s. So I decided to then have ChatGPT-4o write a critique of both exams and suggest areas for improvement, as well as speculate more about how well each “student” (aka ChatGPT-4) did. So this is more an experiment in grading and rubrics and feedback then anything else, but I’m just wanting to explore this somewhat.
Question 1 prompt: I am writing an exam for a causal inference class. Create a question about difference in differences asking them to analyze and interpret Stata output and betas and standard errors.
TEST 1
GPT-4 produced answer in March 2023:
In a causal inference class, you have learned about the difference-in-differences (DiD) estimator as a method for identifying causal effects in observational data. In this question, you are provided with STATA output from a DiD analysis that investigates the effect of a policy intervention on the income of individuals in treatment and control groups, before and after the policy was implemented. Analyze the STATA output and interpret the betas and standard errors.
Consider the following STATA output:
Based on the provided STATA output, please answer the following questions:
What is the estimated causal effect of the policy intervention on income, as measured by the DiD estimator? What is the standard error of this estimate?
How can you interpret the coefficients and standard errors for the 'treat', 'post', and '_cons' variables?
Is the estimated causal effect statistically significant at the 5% level? Explain your answer.
Based on the R-squared and adjusted R-squared values, how well does the model fit the data?
What assumptions are necessary for the validity of the DiD estimator in this context? Briefly discuss if these assumptions might hold in this case.
Answer DiD prompt: Answer the difference-in-differences question that you wrote.
The estimated causal effect of the policy intervention on income, as measured by the DiD estimator, is the coefficient of the interaction term 'treat#post'. The estimate is 4,535.4, with a standard error of 912.7.
The coefficients and standard errors for the 'treat', 'post', and '_cons' variables can be interpreted as follows:
'treat': The average difference in income between the treatment and control groups before the policy intervention, holding other factors constant. The estimated difference is -1,020.8, with a standard error of 846.5.
'post': The average change in income for the control group after the policy intervention, holding other factors constant. The estimated change is 1,010.1, with a standard error of 827.3.
'_cons': The intercept term, representing the average income of the control group before the policy intervention, holding other factors constant. The estimate is 30,781.1, with a standard error of 601.7.
The estimated causal effect is statistically significant at the 5% level, as the p-value for the interaction term 'treat#post' is 0.000, which is less than 0.05.
The R-squared value is 0.2718, and the adjusted R-squared value is 0.2694. This means that approximately 27% of the variation in income can be explained by the model. The model's fit is not very strong, but it does provide some explanatory power.
The key assumptions for the validity of the DiD estimator in this context are: parallel trends (in the absence of the policy intervention, the treatment and control groups would have followed the same trend over time), no spillover effects (the treatment does not affect the control group), and stable composition (the composition of treatment and control groups remains stable over time). In this case, without additional information on the context and data, it is challenging to assess whether these assumptions hold. One would need to investigate the pre-intervention trends, potential spillovers, and the stability of the groups to ensure the validity of the DiD estimator.
Next I asked CHatGPT-4o to grade the solution he provided 14 months ago, as I’m curious a little what, if anything, improved. Interestingly he started out with a rubric. I told him a student took this, not ChatGPT-4.
ChatGPT-4o grade:
Grading Criteria:
Identify the estimated causal effect and standard error (2 points)
Correctly identifies the coefficient of the interaction term 'treat#post' as the DiD estimator (1 point).
Correctly states the standard error of the interaction term (1 point).
Interpretation of coefficients and standard errors (4 points)
Correctly interprets the 'treat' coefficient and standard error (1 point).
Correctly interprets the 'post' coefficient and standard error (1 point).
Correctly interprets the '_cons' coefficient and standard error (1 point).
Clarity and correctness in explanation (1 point).
Statistical significance (2 points)
Correctly determines if the estimated causal effect is statistically significant at the 5% level (1 point).
Explains the reasoning based on p-value (1 point).
Model fit (2 points)
Correctly interprets the R-squared value (1 point).
Correctly interprets the adjusted R-squared value (1 point).
Assumptions for DiD validity (4 points)
Lists and explains the key assumptions (2 points).
Discusses the likelihood of these assumptions holding in this context (2 points).
Student's Answers Evaluation:
Question 1:
Correctly identifies the coefficient of the interaction term 'treat#post' as the DiD estimator: 1 point
Correctly states the standard error of the interaction term: 1 point
Score: 2/2
Question 2:
Correctly interprets the 'treat' coefficient and standard error: 1 point
Correctly interprets the 'post' coefficient and standard error: 1 point
Correctly interprets the '_cons' coefficient and standard error: 1 point
Clarity and correctness in explanation: 1 point
Score: 4/4
Question 3:
Correctly determines if the estimated causal effect is statistically significant at the 5% level: 1 point
Explains the reasoning based on p-value: 1 point
Score: 2/2
Question 4:
Correctly interprets the R-squared value: 1 point
Correctly interprets the adjusted R-squared value: 1 point
Score: 2/2
Question 5:
Lists and explains the key assumptions: 2 points
Discusses the likelihood of these assumptions holding in this context: 2 points
Score: 4/4
Total Score:
14/14
The student has provided comprehensive and accurate answers to all the questions, demonstrating a clear understanding of the DiD estimator and the interpretation of the STATA output. The responses are detailed and correctly address each part of the question.
Additional Comments:
The student's interpretations are clear and well-articulated, providing an accurate analysis of the STATA output.
The explanation of assumptions and the discussion on their validity show a good grasp of the underlying concepts necessary for causal inference using DiD.
TEST 2
Now let’s look at what ChatGPT-4o wrote in response to the same prompt.
Stata output looks similar, but interestingly, ChatGPT-4o is using some kind of indenting that it had not done before. Now let’s see the questions.
In the table above:
post
is a dummy variable equal to 1 for observations after the training program and 0 otherwise.treatment
is a dummy variable equal to 1 for companies in the treatment group and 0 otherwise.post_treatment
is an interaction term betweenpost
andtreatment
.
Tasks:
Identify and explain the coefficients of
post
,treatment
, andpost_treatment
. What do each of these coefficients represent in the context of a Difference in Differences analysis?Interpret the coefficient of the
post_treatment
variable. What does this coefficient tell us about the effect of the training program on employee productivity?Discuss the statistical significance of the results. Which coefficients are statistically significant, and what does this imply about the training program's impact? Use the standard errors and t-values provided to support your answer.
Calculate the total estimated effect of the training program on employee productivity for companies in the treatment group after the program was implemented. Show your calculations.
Critically evaluate the model's fit. What does the R-squared value indicate about the explanatory power of this model?
Suggest one potential limitation of using the Difference in Differences method in this study. How might this limitation impact the results?
Answers:
Explanation of Coefficients:
post
coefficient (0.421): Represents the change in productivity over time for the control group (companies that did not receive the training).treatment
coefficient (0.132): Represents the difference in productivity between the treatment and control groups before the training program was implemented.post_treatment
coefficient (0.298): Represents the additional change in productivity for the treatment group after the training program was implemented, relative to the control group.
Interpretation of
post_treatment
Coefficient:The coefficient of
post_treatment
(0.298) indicates that the training program led to an additional increase in productivity of 0.298 units for the treatment group, beyond the changes observed in the control group over the same period.
Statistical Significance:
The coefficients of
post
(p < 0.000),treatment
(p = 0.033), andpost_treatment
(p < 0.000) are all statistically significant at the 5% level. This implies that the observed effects are unlikely to be due to random chance, and the training program likely had a meaningful impact on productivity.
Total Estimated Effect Calculation:
Total effect for the treatment group after the program =
treatment
+post_treatment
Total effect = 0.132 + 0.298 = 0.430
Model Fit:
The R-squared value (0.0564) indicates that approximately 5.64% of the variance in employee productivity is explained by the model. This suggests a relatively low explanatory power, meaning that other factors not included in the model may also significantly influence productivity.
Potential Limitation:
One potential limitation of the DiD method is the assumption that, in the absence of the treatment, the treatment and control groups would have followed parallel trends. If this assumption is violated, the estimated treatment effect may be biased. For instance, if the treatment group was already on an upward trend in productivity relative to the control group before the training program, the DiD estimate might overstate the true effect of the training program.
ChatGPT-4o grades this student who took test 2. And by student, I mean ChatGPT-4o a half hour ago. Again it designed its own rubric.
Grading Criteria for Test 2:
Identify and explain the coefficients (3 points)
Correctly identifies and explains the coefficient of
post
(1 point).Correctly identifies and explains the coefficient of
treatment
(1 point).Correctly identifies and explains the coefficient of
post_treatment
(1 point).
Interpretation of the post_treatment coefficient (2 points)
Correctly interprets the coefficient (1 point).
Provides clear and accurate explanation (1 point).
Statistical significance (3 points)
Correctly identifies which coefficients are statistically significant (1 point).
Explains the statistical significance based on p-values and t-values (2 points).
Total estimated effect calculation (2 points)
Correctly calculates the total effect (1 point).
Shows clear calculations (1 point).
Model fit (2 points)
Correctly interprets the R-squared value (1 point).
Provides a reasonable evaluation of the model's explanatory power (1 point).
Potential limitation (3 points)
Identifies a relevant potential limitation of the DiD method (1 point).
Explains how this limitation might impact the results (2 points).
Student's Answers Evaluation:
Question 1:
Correctly identifies and explains the coefficients of
post
,treatment
, andpost_treatment
:post
: 0.421, change in productivity over time for the control group.treatment
: 0.132, difference in productivity between the treatment and control groups before the training program.post_treatment
: 0.298, additional change in productivity for the treatment group after the program relative to the control group.Score: 3/3
Question 2: 2. Correctly interprets the coefficient of post_treatment
:
post_treatment
: 0.298, additional increase in productivity for the treatment group beyond changes observed in the control group.Score: 2/2
Question 3: 3. Correctly identifies statistically significant coefficients and explains the statistical significance:
Coefficients of
post
(p < 0.000),treatment
(p = 0.033), andpost_treatment
(p < 0.000) are statistically significant.Explains that the observed effects are unlikely to be due to random chance.
Score: 3/3
Question 4: 4. Correctly calculates the total estimated effect:
Total effect =
treatment
+post_treatment
= 0.132 + 0.298 = 0.430.Score: 2/2
Question 5: 5. Correctly interprets the R-squared value and evaluates model fit:
R-squared (0.0564) indicates 5.64% of variance explained by the model.
The model's fit is relatively low, suggesting other factors may significantly influence productivity.
Score: 2/2
Question 6: 6. Identifies and explains a potential limitation:
Identifies the parallel trends assumption.
Explains that violation of this assumption may bias the estimated treatment effect, possibly overstating the true effect if the treatment group was already on an upward trend.
Score: 3/3
Total Score:
15/15
The student has provided comprehensive and accurate answers to all the questions, demonstrating a clear understanding of the DiD estimator and the interpretation of the STATA output. The responses are detailed and correctly address each part of the question.
Additional Comments:
The student's interpretations are clear and well-articulated, providing an accurate analysis of the STATA output.
The explanation of the potential limitation shows a good grasp of the assumptions necessary for causal inference using DiD.
Overall, the student has performed excellently on this exam.
Now I asked ChatGPT-4o to grade the exams, reflect on which student seemed to have a better understanding of the material, and then I asked what one exam could’ve done differently to be better.
“Cosmos, could you please now grade the two exams? Which one was better in your opinion? And if one was better, what could the other exam have done to improve? And which student seemed to have a deeper understanding”
Keep reading with a 7-day free trial
Subscribe to Scott's Mixtape Substack to keep reading this post and get 7 days of free access to the full post archives.