Closing out my open browser tabs
Economics, Econometrics, AI, Mike Tyson, Classic Volvos, Teaching and of course Apple Vision Pro
Another day another bunch of browser tabs I have gear up the will, the courage, the fortitude to click the X and close out. Even though in doing so, closing that tab, I might never read that article I was waiting to read, and even though closing out a tab you’ve kept open to read is worse than stepping on a crack, this is part of my journey into self acceptance. So I’ll do my best to try and do that now.
Jake Paul beat Mike Tyson in a unanimous decision last night. Paul is said to have netted $40m and Tyson $20m from it. I tried to watch it on Netflix but never could get on which probably means it was a huge success. Here’s Tyson explaining to a kid that caring about one’s legacy is a form of ego and that all he’s going to be leaving behind is his dead body, not “legacy”. Sounds about right tbh.
Jessica Hoel talks about stagnant faculty raises and growing staff and administration.
The AEA5k tee shirts are out and they look gorgeous. Hats off to them.
Here’s a great article explaining how large language models work without all the jargon.
The history of the “overdrive” on your gearbox. I don’t know if it’s safe to close this tab actually because I think there’s a chance I may need to learn about the history of the overdrive so I may wait. I wonder if I could change the rules to where I have to close all the tabs but can technically keep two.
This cherry red 1970 Volvo 1800E on bringatrailer is being sold with no reserve. It was sold in 2023 for $19k but the owner and buyer “mutually” agreed to dissolve the sale after the auction. I watch it every day. It’s got three days left. And yes it has an overdrive. Maybe every car has an overdrive though; I never read that article so I don’t know.
Here’s a 1985 Volvo 240 with an overdrive too. Subscribing to all the Volvo auctions on bringatrailer has had its pros and cons.
Google news sends me stuff it knows I am curious about but don’t actually need to know since I’m not dating. But I read this stuff anyway. The practice of ghosting is where a person abruptly stops talking — I figured that one out. But then there’s something called “zombie-ing” where they show back up after ghosting like six months later. It’s supposedly far worse. But Mike Tyson, recall, said we all are going to die one day anyway, so let’s have some perspective. Maybe dating someone who believes our legacy matters is worse than zombie-ing.
Briefly, the real o1 got out into the wild — o1 being the real version of our current o1-preview. Not sure what the ruling on it was. I realized though in writing this that maybe o1-preview could be my answer to having trouble with ChatGPT understanding what I needed on making tikz graphics in latex. But o1-previews takes sooo long. Anyway, wish me luck to figuring out all those questions.
Tips on using ChatGPT Search more effectively. Search is the new search engine button on ChatGPT. I use it constantly but also when I don’t think that ChatGPT maybe has the most up to date info so I just ask the same question with Search turned on just to see.
The new M5 chip for Apple Vision Pro is still planned for 2025. Though reports have come in that they also have stopped production on the Apple Vision Pro line. I just hope we get the new visionOS update soon with the gigantic virtual monitors. Although I guess there’s worse things than not getting gigantic virtual monitors for your Apple Vision Pro such as believing in one’s legacy or getting zomebied or being the zombie.
Here’s the Southern Economic Association’s conference schedule if you’re interested.
Carolina Cattaneo and Brantly Callaway on diff-in-diff models in which parallel trend holds only after conditioning on covariates.
Here’s an internship option for people interested in causal inference and experimentation at Netflix.
Tutorial on using matrix completion with Yiqing Xu’s gynsth package in R. Here’s Susan Athey’s original MCPanel GitHub repository, though, for it.
Doudchenko and Imbens 2017 article on synthetic control was, I think, Imbens foray into studying the properties of synth and extending it. It was never published, but the ideas in it ended up in other papers he’d write on it, such as relaxing the convex hull with an intercept term, negative weighting, reframing synth as a vertical regression. Either I’m right about that, or I just can’t keep it all straight.
I’ve been going through dissertations in my downtime the last week. Here’s Andrew Goodman-Bacon’s dissertation. And here is Angrist and Heckman’s acknowledgments page of their dissertations.
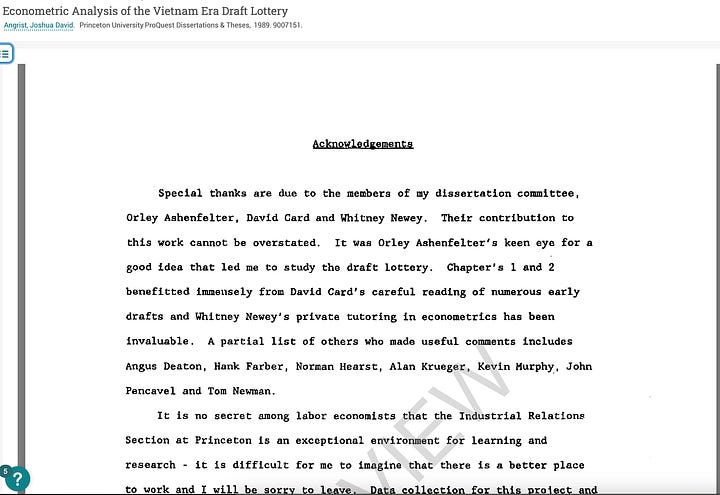
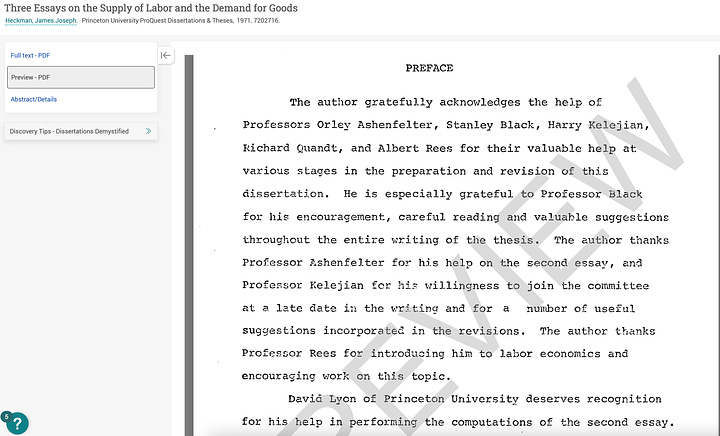
Kyle Butts has a nice blog post explaining what factor models are. They are very common in synthetic control, so this is very helpful.
Abadie and L’Hour have a paper on synthetic control for disaggregated data in which there are multiple treated units. They note that building a separate synth for each treated unit can be a way to avoid interpolation biases, but the problem is that there is not a unique solution. There’s various types of biases that creep up in that context, and they propose an estimator that penalizes “pairwise discrepancies” the observable imbalance between the treated and synth pairs. The penalization trades off the matching bias with respect to the individual units in the synthetic control and the overall aggregate synthetic control. And they propose data driven choices for the penalization. The podcast hosts at NotebookLM’s “deep dive podcast” can break it down for you.
AI and its role in scientific discovery by Aidan Toner-Rodgers, a PhD student at MIT economics. Toner-Rodgers explores how AI impacts innovation in a U.S. materials science R&D lab. Using a randomized rollout of an AI-powered materials discovery tool to 1,018 scientists, he finds big gains: a 44% boost in materials discovered, 39% more patents filed, and a 17% jump in product innovations. The tool, powered by graph neural networks, enables “inverse materials design”—input desired properties, get predicted compound structures. These AI-generated compounds are more novel and lead to radical inventions.
But, there’s inequality among workers in how these discoveries materialized, and it’s not quite what we’ve seen before in terms of how AI impacts the returns to experience and skill. Here, Toner-Rodgers finds that top researchers nearly double their output, while less productive scientists see little improvement.
So, why does this happen? The AI excels at generating ideas, which already I think is something people have been skeptical about, but ideas do not itself lead to success. Success depends on spotting the most promising leads—a skill top scientists leverage better. Surprisingly, 82% of scientists report lower job satisfaction, citing reduced creativity and underused expertise. The study highlights both the promise and the challenges of AI-augmented research.
Fascinating study — best one I’ve read on AI by an economist in a while. Here’s the notebookLM hosts talking about the paper.
Wenjia Cao is a PhD student at Michigan State University and she is also studying AI and labor questions. She’ll be presenting at the Southerns in one of my sessions.
Paul Samuelson, Summer 2004 Journal of Economic Perspectives, on Ricardo and Mill’s response to then mainstream economists support of globalization. I wonder if reading it and reading Autor, Dorn and Hanson’s 2013 AER, “China Syndrome”, would make for interesting comparisons.
Jim Heckman introduces Becker’s theory of time allocation study in a 2015 EJ.
This week I gave a talk to our business school about using AI for research.
Here’s the pdf of the talk if you want to see it. I first framed the whole thing in terms of two things:
Becker’s theory of human capital. I emphasize that to get the most out of AI tools, you have to develop skills and human capital, which largely will come through intensive time use and experimentation.
Becker’s theory of the allocation of time use. I emphasize that humans make things through human exertion which involves labor with tools to turn raw materials into output. And gen AI allows you to complete tasks using fewer time inputs.
Since you use less time, there’s two effects we can expect at minimum in that neoclassical framework:
Output effect. We can expect that holding constant the time spent on the task, you get more done.
Substitution effect. We can expect that since the time necessary to complete the task has fallen, you will shift towards other activities.
But then I also note the potential problems that gen AI creates for productivity through automation. I give examples of that such as sunk cost fallacies, which I’ve not seen people talk about before. But an example of it comes with coding where the coding you engage in using gen AI becomes very complex, but then you may find the gen AI gets “stuck”. But since your human capital is only bound up in “prompt coding”, you may find you cannot solve the problem, nor can your AI chatbot. Rather than start over yourself, you may just keep trying and getting nowhere, which is one version of a sunk cost fallacy using gen AI for coding.
It’s a talk about “practical” things for research, so I give a lot of advice of ways to work around that, such as what I call “poor man’s mixture of experts” where you switch between chatbots quickly when you feel stuck. I break it down into practical suggestions on topics of “time use” and “human capital”:
Time use stuff
“Personalized” citation search, literature review, summaries using o1-preview in combination with ChatGPT-Search
Stuff on sunk cost fallacies
Human capital stuff
Conversational prompting, as opposed to barking orders
NotebookLM overviews of difficult new papers to get a map
Poor Man’s Mixture of Experts and “Orthogonal errors” across LLMs
Covariate and instrumental variables search
But I basically try to emphasize that automation has an ambiguous effect on productivity — Ricardo wrote about this in his 3rd edition of Principles and Samuelson has as well, as has Acemoglu. I try to emphasize also that there is a situation where the substitution effects will increase your overall output, but I also say that that could be masking a kind of “pure automation” in which you actually end up making less, and that the substitution effect and the automation effects might be hard to discern until later, which is why it’s helpful to think of it up front.
Basically, what I’ve been telling my students is that they have to use AI to enhance their time use, make themselves more productive in their time use, and continue to use the same amount of time as before. It’s not that substitution effects are not desirable — they are. I just think that the risks of completely shirking from the tasks altogether because you believe you’re downloading knowledge at some hyper speed directly into your brain is very high. Ultimately, my hunch is that you learn things via intensive time use, and if you actually reduce your time investments in learning, then you will learn less, period. Obviously if each unit of time is more productive towards the task of increasing human capital, then you technically can reduce time and learn more, but my point is that I bet you that more of the “automated learning but not learning anything” equilibrium is happening than that, and when that even is happening, I bet it’s happening because a person is well aware of the hazards and has a strategy for navigating them.
Here is something I wrote up for my students, btw, to help them use AI tools to study for our final exam as well as to help them come up with the thesis for their final essay. The course prohibits AI to write for this assignment, and it’s an honor code so I’m trusting them (it works both ways) to comply. But I want them to use AI this semester in a creative way, to brainstorm and focus their time. Though as Toner-Rodgers said, that substitution away from creativity in a science lab actually led to dramatic reductions in job satisfaction precisely because it caused a substitution away from personal creativity. Question is whether there are heterogenous treatment effects — that may not be generalizable to every type of worker, including students, or faculty, but it’s enough of an issue that we should be studying it.
But that aside, here is the explanation to my students about using AI to help them wrap up the semester.
And that’s it! Now I have to get ready for a workshop — two days of synthetic control! Wish us luck as we use our time and human exertion to build knowledge and increase aggregate output!